Interview: Valeo advances self-driving vehicle development in Czechia
A self-driving car is a big computer on wheels that has to process a number of tasks every second. That's why it takes years to develop a new system and put it into practice. Everything must work flawlessly and reliably. We talked to David Hurych and Jakub Černý of Valeo, experts in the application of artificial intelligence systems to self-driving units, about the development and future of the automotive industry.
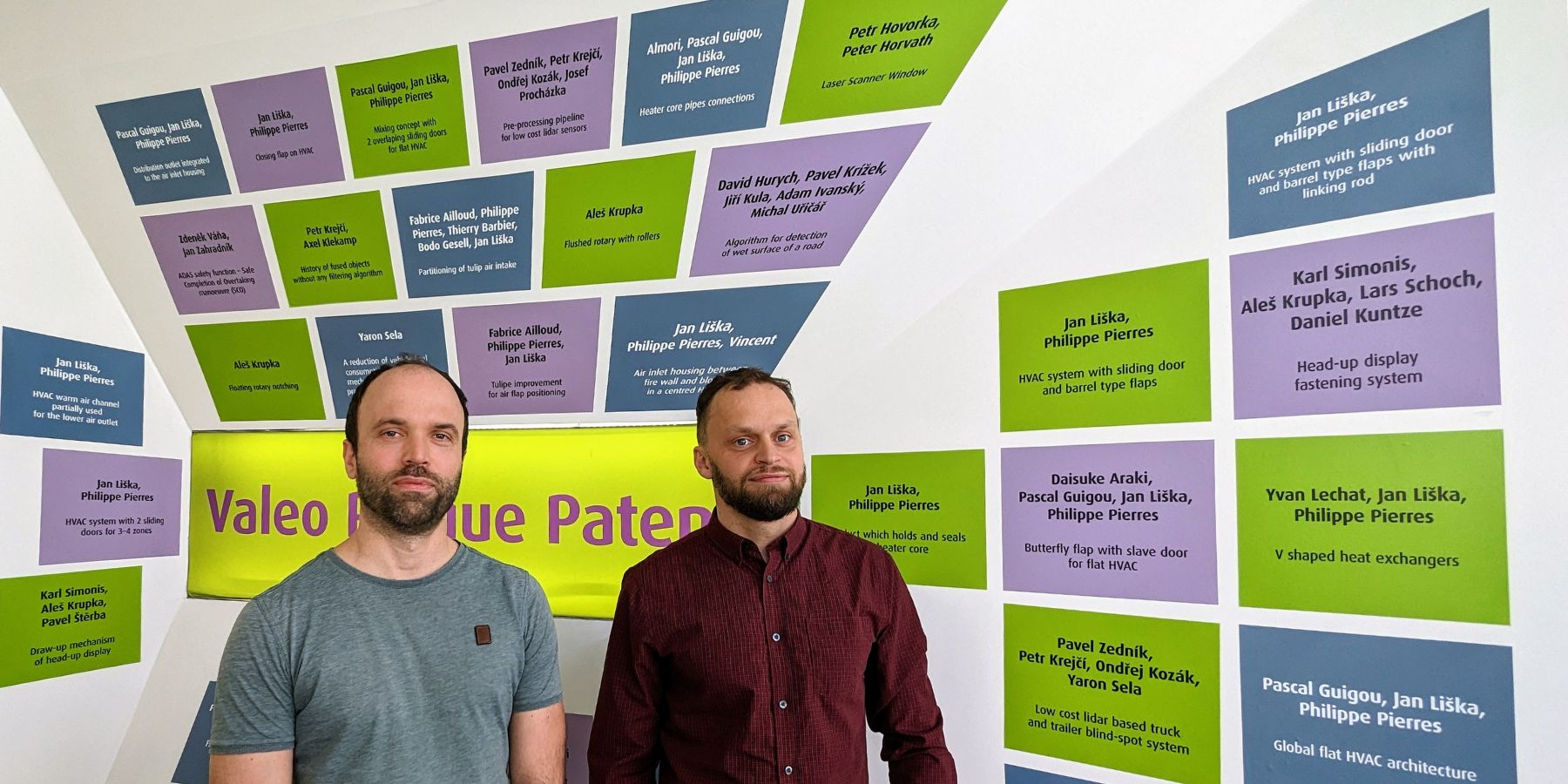
Valeo is a world leader in automated vehicle systems, with one in three new vehicles currently equipped with its technology. By 2025, 75% of new vehicles will be equipped with advanced active safety systems. These will allow the vehicle to accurately monitor its environment and trigger safety manoeuvres such as automatic emergency braking. In the race for leadership in driving assistance systems, Valeo has already made history. The first two cars to achieve Level 3 autonomy have entered the market: the Honda Legend and the Mercedes S-Class. Both models have one thing in common – they are equipped with LiDAR technology, the Valeo Scala® sensor, the first mass-produced lidar sensor on the automotive market.
How would you describe your job to a five-year-old?
David Hurych: We are making cars safer so there are fewer accidents, fewer deaths and people can rest in their cars.
Jakub Černý: I improve people’s jobs so that they don’t have to do repetitive activities and can do things with more added value and more interesting work.
Can you explain what Valeo Prague is doing in the field of artificial intelligence?
DH: The annotated validation data is also used to run the built assistance system and compare its decisions with the annotations. In this way, we are able to validate the accuracy of these systems before they are used in real-life situations. A large amount of annotated validation data is required. Level 3 autonomous driving already requires around 33,000 hours of driving with a system. And every second, every frame needs to be annotated. It’s a mammoth task, billions of images.
What is this validation for and why is it important?
DH: Validation is important because we need to know what the system will do on a large statistical sample of data.
The difference with testing on available datasets in an academic environment is that we have to work in the real world – at night, in a tunnel, in the rain, outdoors, in the Alps, in fog. There are an almost infinite number of possibilities that we cannot see and cover. So validation is very important with this huge amount of data, because we try to capture as many different possible situations as possible to make sure that the whole system is really reliable. To oversimplify it, it’s about being able to predict how the car will behave in every possible situation. We’re never going to have a pink inflatable elephant in front of the car – because we’re not going to record that unless we put it there ourselves – but we try to cover as wide a range of possibilities as realistically as possible.
Then there are other validations with prescribed scenarios. You’re trying to park to the right – there’s a car in front of you, there’s a pedestrian behind you, what’s the system going to do? The scenarios are prescriptive, so in this case it’s not directly statistical validation, but examples that we’ve come up with together with customers that make sense to us.
About how many sensors are there in the car?
DH: It varies. Every car has something different, depending on the number of systems and the customer’s choice. An extreme example would be our Drive4U prototype, a proof of concept for building a Level 4 autonomous driving system with mass-produced sensors. This has 6 LiDARs, 4 fish-eye cameras, 1 front camera, 12 ultrasonic sensors and 4 radars. In total, that’s over 20 different sensors. But this is really a proof of concept, the computing power is provided by a separate powerful computer in the boot of the car.
Level 3 self-driving systems are “eyes off” conditional automation systems. In a defined environment, the driver does not need to take over the wheel, but must be ready to do so within a time limit set by the manufacturer. In Europe, Level 3 is only permitted in Germany on approximately 13 thousand kilometres of highways.

When do you think full-fledged Level 3 self-driving systems will appear on the roads?
DH: Level 3 self-driving systems are already on the roads, for specific situations. For parking, highway driving, that sort of thing. Level 3 systems always serve specific purposes. There is not yet a general Level 3 system that covers all situations.
Does the Czech legislation, for example, provide for such a situation?
DH: It is, as far as I know, very far behind. In the US, they are better off, the Czech legislation is simply not keeping up at all. It is a Europe-wide problem, the environment here is very regulated.
Is there a risk of technology developing faster than legislation?
DH: There is no risk, it is already happening.
What is currently biggest problem of autonomous systems that remains to be solved?
DH: There are too many. For example, right now we’re trying to move away from learning with a teacher towards learning without a teacher so we can get rid of manual annotation. It’s terribly expensive, it demands attention, and it’s a mind-numbing job. Once manual annotation is no longer needed, or only minimally needed, the data alone is enough to train AI. And we are also trying to reduce the volume. It’s not about not having the data, but having it generated, for example. To reduce the need for annotations, we use self-supervised learning methods, which can be thought of as follows. You teach a neural network to orient an image correctly. For example, you deliberately roll it up, give it a scale, and tell it to straighten it. When it learns this on a large amount of data, it learns a lot of very useful features for final detection of people and cars. Then it only needs 10 annotated images instead of five million and it adapts to the task. The “body”, the image processing, has already been learned and therefore it will recognise certain signs elsewhere. Then there are various semi-supervised methods where, for example, only 10% of the annotations are needed instead of 100%.
We also use models that teach each other, such as teacher-student. So we try not to have annotations and try to reduce the need for real data. We’re already able to generate data quite well, but it’s a kind of “which came first – the chicken or the egg? If you want to generate data, you need data to teach the data generator something. We’re trying to reduce the percentage of those real data sets so that we can augment them with virtual data. Text processing also uses supervised learning. For example, you mask the words in a sentence and the neural network tries to fill them in on its own. Even if the final task is something else, such as classifying text data in a table, the model learns the necessary tasks using the self-supervised method, and then simply “bends” to the final task with a minimum of annotated data. So it is a matter of letting the models learn as much as possible on their own and just presenting them with huge amounts of data.
So you have enough data?
DH: In general, yes. In research, you typically work with data sets. What we have here is what I call a pile of data. Making a data set out of a pile of data is a challenge in itself. In the automotive industry, the problem is not that we don’t have the data, but that we don’t have the time to process it. Within a task or a project, we can do it. It’s just that if we want to use it further, we run into a problem of computing power. Recently, as part of a European project, we were able to secure access to a supercomputer for three years, which will give us some relief.
JČ: For us – in manufacturing – the problem is a little different. We have the data, but sometimes we need, for example, to detect a defect in a product that occurs, say, once every 14 days. Then it’s just a few images, and it’s hard to work with that.
Security standards are improving and people’s mindsets will also change over time. It will be a combination of trust and resources. I see the current mistrust. Knowing that an unforeseen event can happen at any time – and I have no idea how the system will react – is a problem. That’s what we’re trying to improve.
With the public, one often encounters a distrust of self-driving cars, a reluctance to let the machine do the driving. Do you see the same problem and do you think that public opinion will change over time?
DH: Yes, I feel the same way, but I’m sure that will change over time. It’s a mindset thing. To get into an autonomous car, the car has to be not as accurate and accident-free as you are, but it has to be much better. You have to really trust the system to be able to take a nap in the car. It’s about trust, and it’s about valid statistics. You need to have the system really tested, you have redundant systems solving the same task in parallel and checking each other.
Safety standards are improving and over time we need to get to a stage where people’s attitudes change and they say it’s not worth it. They will see extremely low accident rates and it will start to make financial sense to buy. It will be a combination of trust and resources. I see the current mistrust and in a way I have it too. I know what to expect from the person, but I don’t know what to expect from the system. Knowing that an unforeseen event can happen at any time – and I have no idea how the system will react – is a problem. That’s what we’re trying to improve, to generate data, to test the maximum number of situations that can ever occur.
At some point there will be a change. Perhaps with the change of generations – with young people who may not even want to get a driving licence – it will be easier to accept autonomous systems. But that’s speculation.
What do you think the future of autonomous driving will be? Will there be more order on the roads? What can we expect?
JČ: I think it can be more efficient than it is now, if you look at the roads here… For example, it bothers me that cars don’t communicate with the intersection. I’m talking about a situation where you come to a junction, you stop at a red light, but no one is coming from the opposite direction. There are proposals for such a solution, even concrete solutions. My automation professor, Mr Laurel, used to say that until this is autonomous, cars will crash.
DH: I agree, although there will always be crashes. But if car manufacturers get their act together and standardise vehicle-to-vehicle communication, the situation on the roads will improve. There will be less congestion because speeds will be optimised and traffic will be smoother. Statistically, of course, the accident rate for autonomous systems will be much lower than the human accident rate. Sometimes accidents will happen – for instance, if a system hits ice in Cairo, you can’t count on that – but I think the future is definitely positive. Maybe it’s a bit negative in the sense that people will get a bit lazy again, they won’t even be able to drive, and there will be one less skill.
If you drive, what do you drive?
JČ: I try to run to work, but otherwise I have a Hyundai i30.
DH: I try to cycle to work. Until a year ago I drove a 1998 Felicia Combi, now I have a Superb, but basically no features. I’d like to have parking systems, sensors and so on – and probably will get them eventually – but I get annoyed by lane keeping and the like, which gets in the way of steering a bit, and often not optimally. Because it’s terribly difficult to set it up for each individual driver. Everyone drives a little differently. If you set it up for the ‘average’ driver, it just doesn’t work very well. There are already systems that adapt to a particular driver’s style. The system first observes how you drive and gradually adapts. It also learns to be more aggressive – for example, if you need to turn left, there’s a queue and nobody wants to let you through. When a human is driving, he starts to push into the traffic a bit. The systems are now doing that too, because otherwise they wouldn’t move out of the way.
Where in the world do you see the main technology hubs in your industry?
DH: China is good at autonomous driving, they’re pushing it a lot and most importantly they can simply give an “order from above”. Europe is very regulated, a bit slower, although the quality is maybe a bit higher. It just seems to me that it’s just coming here with a delay and hopefully higher quality.
JČ: When I look at the Valeo subsidiaries, I think Germany is the best. They have an explicit Advanced Technology department in Germany and they’re doing some really interesting things there. For me, they’re ahead of the curve, and my understanding is that they also have very generous support from the German government, or in this case the Bavarian government. I think there’s a breeding ground there to move towards what’s called Industry 4.0, which is a big mantra, but the reality is a bit different.
Is AI more of a threat to you or are you looking forward to its development?
DH: I don’t really understand all the scaremongering. I understand that if a generator like GPT 4 got into the hands of an outsider, it would be able to generate a lot of fake news very effectively. However, if I were to be afraid of anything, it would be these text generators that can clutter the internet with relatively well-crafted ballast. But other than that, I wouldn’t worry about the evolution at all. A neuron or AI will do exactly what you tell it to do and what you teach it to do. It doesn’t have an agenda or a mind of its own, it needs powerful hardware and a lot of care, it’s fragile. We’re still a long way from Skynet, but what I see as an urgent need is the parallel development of fact-checking and fake news detection systems that allow ordinary people to assess the validity of the information they’re reading or watching on video, so that they’re not easily manipulated.
My dream is that on every website we will find a neural network or artificial intelligence whose code will be known and open, we will know the training data and everyone will be able to look at it. Then on every website there will be a banner that says: So and so much of the information on this website is factually correct, creating a fact check for everyone. I think maybe 85% of the information is completely unverifiable, it is just opinion. That is what I would push for, to make the fact-checking stuff free, but also to make it open so that everybody can see how it works. Otherwise, I think we’re a very long way from something dangerous, because in parallel with dangerous ways of using AI, there will be countermeasures.
JČ: It’s a tool like any other. You can use it for good or evil. I like the saying: Artificial intelligence is no match for natural stupidity. And that still applies.
It is sometimes suggested, even among the more expert community, that AI could have similar consequences to the first industrial revolution. Chaos, lots of people will lose their jobs, they won’t be able to adapt. How do you see it?
DH: It’s going to eliminate a lot of jobs, but it’s also going to create a lot of jobs. I see a lot of new jobs now, like neural network teachers. They will be annotating data and re-teaching the neural networks. Change is definitely coming, that’s true, but I don’t see any reason to panic, on the contrary, it’s an opportunity to do things more efficiently and I see AI as an enabler.
JČ: I totally agree. We see it in practice. We go into manufacturing plants where we take the work of half an operator. We have just added a new one for the other two. As my colleague said, someone else, usually a more educated person, has to be there to annotate the data. They have to know what they’re doing. If they make a mistake, they’re in more trouble than if they usually just make a bad part. And we certainly can’t replace operators in a year or two – it’s a long run.
DH: One day there may not be a need for an operator, but the transition will not be a leap, it will take years.
Generative Adversarial Network (GAN) is a machine learning (ML) model in which two neural networks compete with each other using deep learning methods to make their predictions more accurate.
How do you use GANs at Valeo in your work?
DH: Valeo uses GANs in two ways.
Imagine a picture where instead of a car, there is a blue blob with the exact contour of the car. Next to that is a green blob – a tree. It’s a semantic map that tells you where to put what class. This is the pixel class car, this is the class tree. You can generate the semantic map easily. We have that as an input and we can get a real image from that with the help of a neural network. We’ll build a scene and with some quality we’ll get an image out of it. So we can build a scene where a person is standing on top of a car and things like that. It’s not perfect, but that kind of generated data is very helpful in learning. We are working on being able to generate this data for testing and system validation. That’s more challenging because we can’t have artifacts in there. It’s also about image quality, plus the scene must not be too far from reality.
The other application of GANs that we use is for example inputting people. The input image is a scene, and we say we want to draw a person in that position. We choose a position and it plots it there. We can say that we are able to extend our set of images with people. That way we have automatic annotation. We know where the person is and we can continue to learn models based on that info. By generating people in different scenes, we can improve our detection for the car. Very specifically, this has helped in urban scenes during the night where we’ve gotten much better detection accuracy. This is just one example of the use of GANs.
David Hurych has been with Valeo for 10 years. Prior to joining the company, he earned a PhD at the Department of Cybernetics at CTU – majoring in Biocybernetics and Artificial Intelligence. He joined the company as a Research Engineer and SW Team Leader, where he and his team were tasked with learning neural networks and other AI models for automotive use.
For the last 4 years, he has been a Research Scientist in the Valeo AI team. The team is mostly based in Paris, with over 20 members in total, including two in Prague. He is dedicated to research and publishing on state-of-the-art deep neural networks. David is also in charge of supervising PhD students.
Jakub Černý has been with Valeo for four years. He is part of the newly created department that deals with industrialisation. The task of the department is to link the R&D and the production parts of the company. It focuses on the application of AI technologies for industrialisation directly in production lines.
He graduated from Brno University of Technology, specifically Cybernetics and Measurement Systems, with a specialisation in image processing. At Valeo, he supports the entire team in the creation and practical application of AI models.
Watch the test-drive of Valeo’s demonstration car in the heart of Paris.
Next up from prg.ai
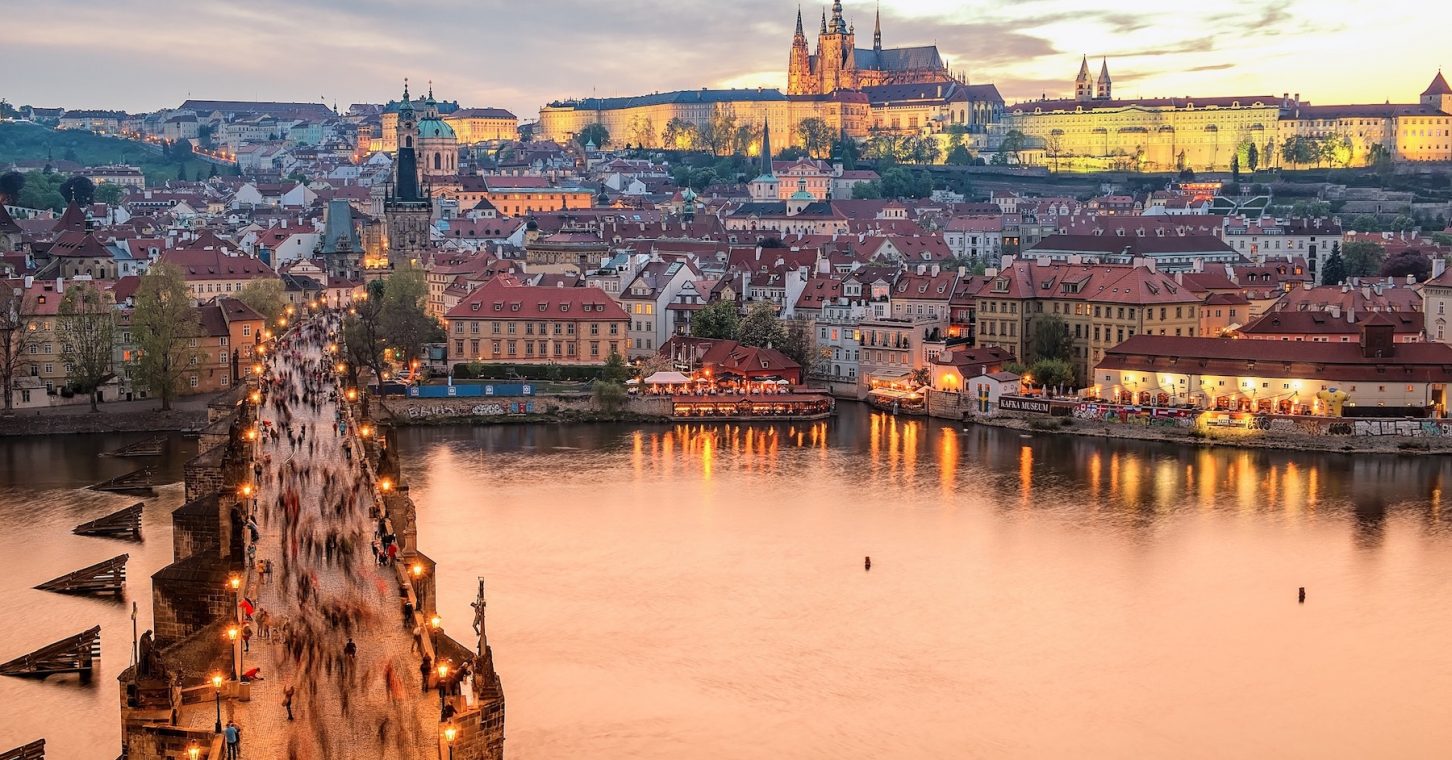
Typical Prague AI firm is young, self-sufficient, and export oriented, shows our new comprehensive study
130 companies, 11 interviews, 9 business topics. Explore all that and more in the unique study authored by prg.ai, which contains an overview of last year's most notable events on the local AI scene or articles on the future of AI or gender equality in research.
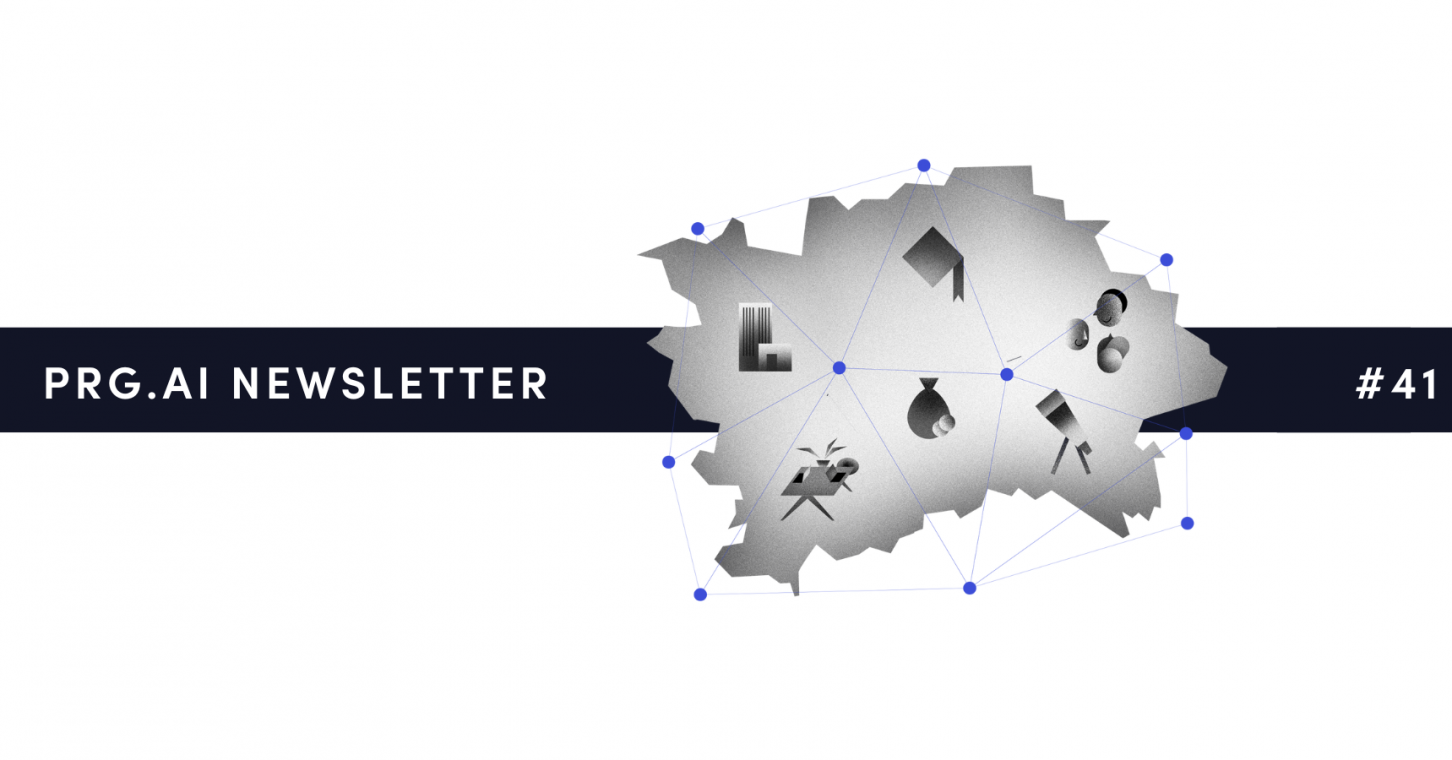
prg.ai newsletter #41
The first spring edition of our newsletter! Get the latest prg.ai updates, exciting news from the Prague AI scene, a curated list of interesting events, open positions, and much more. Stay in the loop!
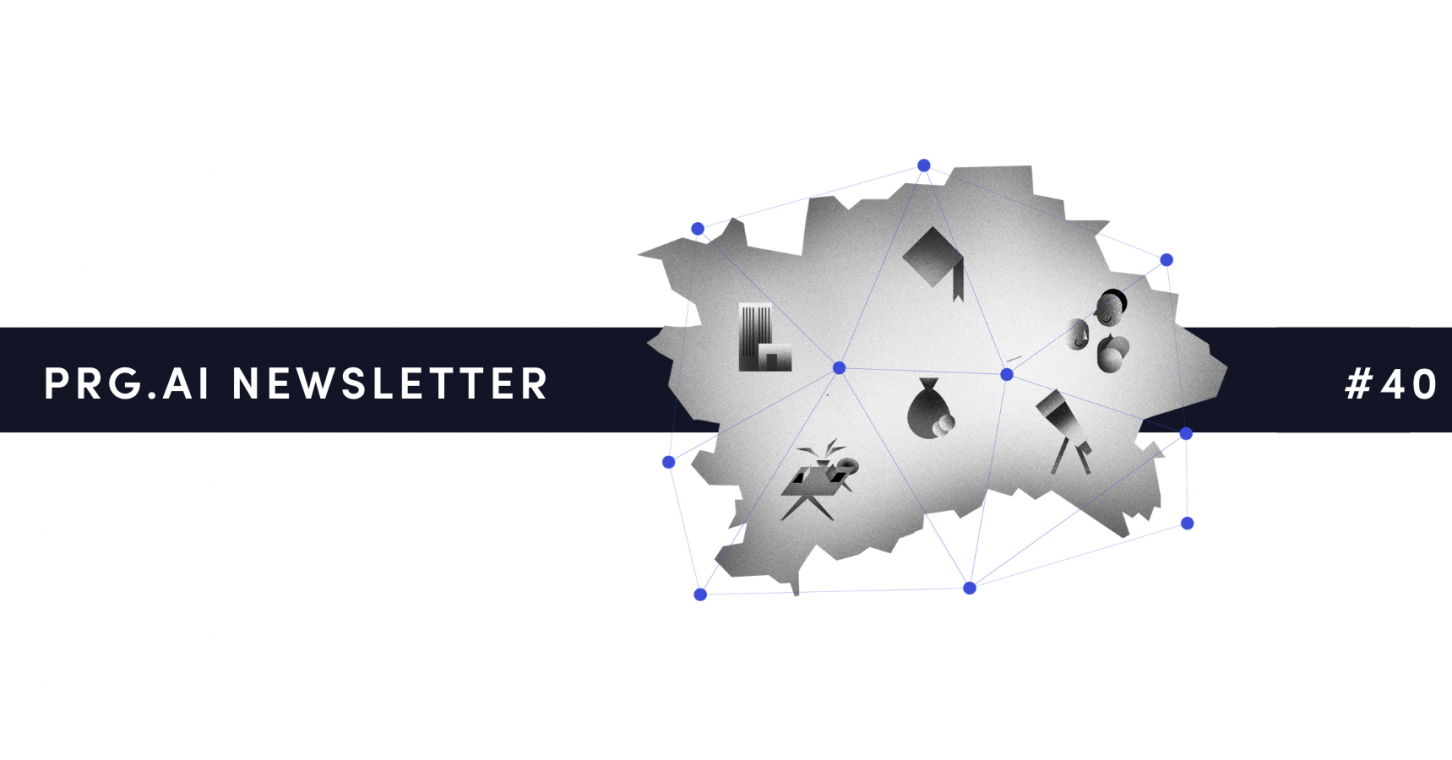
prg.ai newsletter #40
The fortieth milestone issue of the prg․ai newsletter is packed with news and intriguing facts not only from the Prague AI scene. Keep reading so you don't miss out on anything!
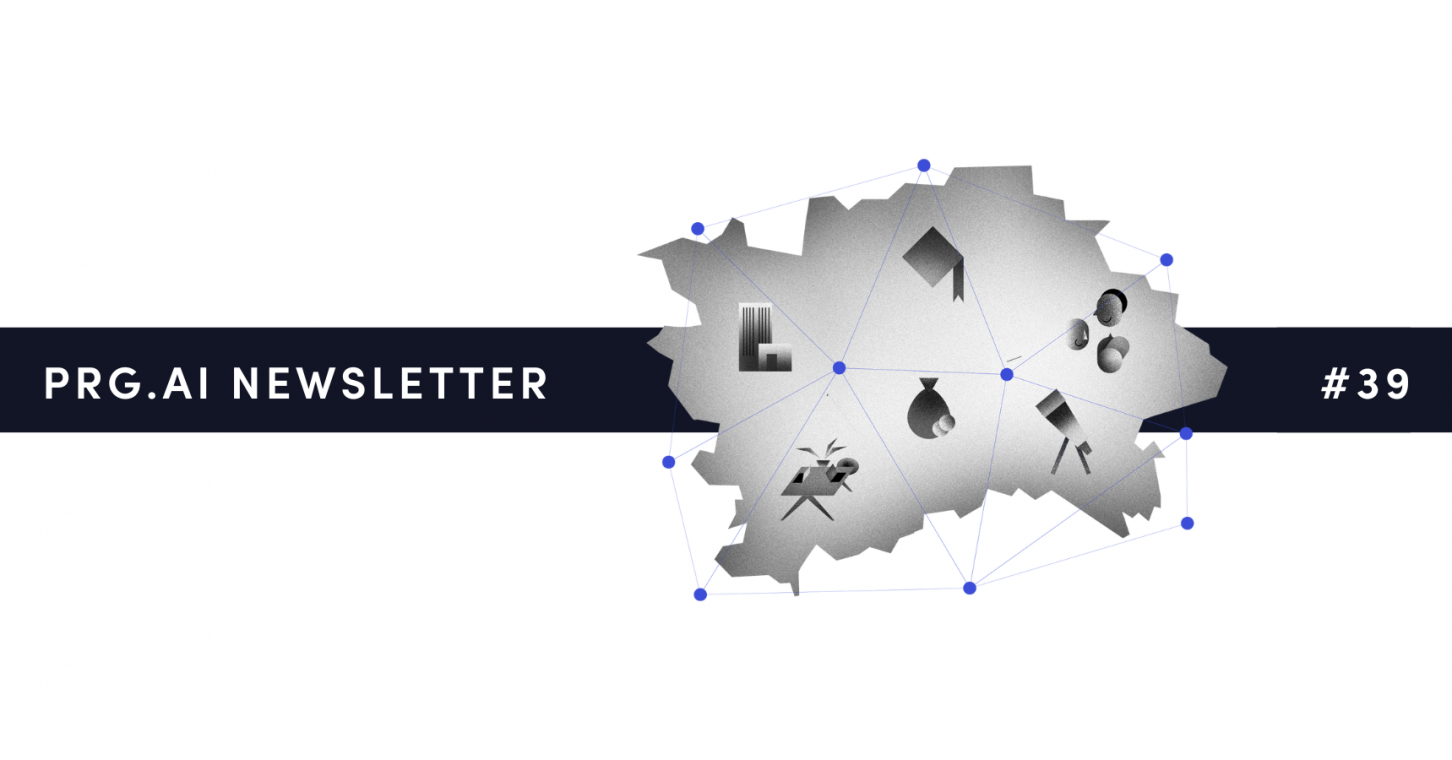
prg.ai newsletter #39
What did the first month of 2024 bring, and what can you look forward to in February? Find out in the next prg.ai newsletter. Check out what's new on the artificial intelligence scene (not only) in Prague.